How AI reshapes predictive maintenance in manufacturing
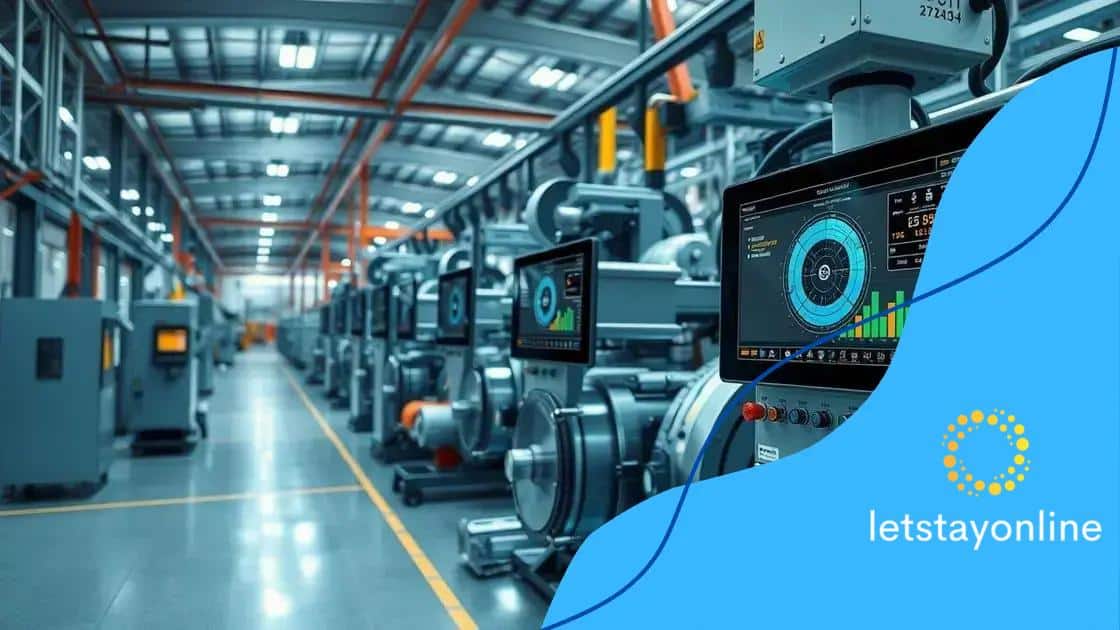
How AI reshapes predictive maintenance in manufacturing by analyzing data to predict equipment failures, reducing downtime and costs while improving overall efficiency and safety in operations.
How AI reshapes predictive maintenance in manufacturing is a game-changer for industries aiming to boost productivity and minimize downtime. Have you ever wondered how machines predict failures before they happen? Let’s dive in.
Understanding predictive maintenance
Understanding predictive maintenance is essential for modern manufacturing. This concept refers to techniques that allow maintenance to be performed just before equipment failures occur. It helps reduce unexpected downtimes and saves costs.
What is Predictive Maintenance?
Predictive maintenance uses data analysis tools and techniques to detect anomalies in your operations. This allows you to predict equipment failures before they happen. By implementing predictive maintenance, companies can avoid unnecessary maintenance tasks while minimizing potential failures.
Benefits of Predictive Maintenance
- Increased equipment lifespan
- Reduced maintenance costs
- Improved operational efficiency
- Enhanced safety standards
Businesses that harness predictive maintenance can monitor the performance of their equipment in real-time. This constant observation offers valuable insights into how machinery operates. Moreover, organizations can act on this data quickly to address issues before they escalate.
Additionally, technology such as IoT devices and machine learning algorithms amplifies the effectiveness of predictive maintenance. These technologies analyze historical data to find patterns that indicate potential failures. It’s fascinating how quickly these predictions can save both time and money.
Implementing Predictive Maintenance Strategies
Implementing a predictive maintenance program can seem daunting, but it begins with collecting data. Machines equipped with sensors gather information regarding performance and conditions. Next, businesses need to analyze this data to enhance decision-making processes.
Establishing a predictive maintenance framework involves several vital steps:
- Identifying critical machinery to monitor.
- Setting up data collection systems.
- Creating baseline performance metrics.
- Incorporating analysis tools for real-time insights.
By doing so, companies can anticipate possible failures and reduce maintenance response times. Transitioning to predictive maintenance fundamentally shifts how organizations view their operations.
In essence, understanding predictive maintenance equips manufacturers with the tools to optimize their processes. By embracing this forward-thinking approach, businesses are better prepared to face the challenges of today’s competitive environment.
The role of AI in predictive maintenance
The role of AI in predictive maintenance is pivotal for enhancing efficiency in manufacturing processes. AI technology enables organizations to analyze large volumes of data quickly. This analysis helps companies foresee equipment failures, optimizing their maintenance strategies.
Artificial Intelligence uses algorithms that learn from historical data to identify patterns. By integrating AI into maintenance systems, businesses can efficiently predict when a machine is likely to fail. This proactive approach can significantly lower repair costs and reduce unplanned downtime.
How AI Enhances Predictive Maintenance
The integration of AI into maintenance allows for better decision-making. Some key benefits include:
- Real-time monitoring of equipment condition
- Automation of data collection processes
- Advanced analytics for insights on machine performance
- Timely updates and alerts regarding maintenance needs
With real-time data, organizations can monitor their assets continuously. AI systems can process information from sensors, enabling timely insights that prompt necessary actions before an issue escalates.
Furthermore, using AI facilitates the creation of predictive models that improve over time as they ingest more data. This self-improving capability means the predictions become more accurate, allowing for better resource allocation and increasing operational uptime.
Challenges and Considerations
While incorporating AI into predictive maintenance offers numerous benefits, there are challenges to address. Initial implementation costs can be high, and there may be a steep learning curve for staff.
Moreover, ensuring data quality is crucial for the success of AI models. Poor data can lead to inaccurate predictions, which could hamper operational efficiency. Businesses need to invest in proper data management strategies to support their AI systems.
In transitioning to AI-driven maintenance, companies must adopt a culture of continuous improvement and adaptability. Embracing change is necessary to fully harness the potential of AI technologies.
Benefits of AI-driven predictive maintenance
Benefits of AI-driven predictive maintenance are changing how businesses operate in the manufacturing sector. By leveraging AI technologies, companies can maximize efficiency and minimize unexpected equipment failures.
One of the key advantages is the reduction of maintenance costs. Predictive maintenance helps companies know exactly when a machine needs attention, thus avoiding unnecessary maintenance tasks. This approach saves time and money while ensuring that equipment runs smoothly.
Key Advantages of AI-Driven Predictive Maintenance
Here are some notable benefits:
- Increased equipment reliability and lifespan
- Enhanced productivity and operational efficiency
- Reduced downtime due to unexpected failures
- Improved safety for employees
Utilizing AI can lead to improved reliability of machinery. When equipment is serviced based on actual needs, rather than on a fixed schedule, performance increases. This means that machines can work for longer periods, maximizing productivity.
Another significant benefit is improved safety. With predictive maintenance, potential failures can be identified and addressed before they pose risks to workers. This proactive approach fosters a safer working environment.
Data-Driven Insights
AI systems provide data-driven insights that help in making informed decisions. Businesses can monitor their equipment in real time, allowing them to react quickly to any issues that arise. These insights not only help in solving problems but also aid in planning for future needs.
The data collected through AI analytics can uncover patterns and trends in equipment performance. By understanding these patterns, organizations can optimize their maintenance schedules and strategies. With this knowledge, companies can make adjustments that lead to even greater efficiencies.
Furthermore, AI-driven predictive maintenance fosters a culture of continuous improvement. By focusing on data and outcomes, organizations can adapt their strategies based on what works best for their operations. It creates a feedback loop where improvements can constantly be made.
Challenges in implementing AI solutions
Challenges in implementing AI solutions in predictive maintenance can be significant. While AI offers numerous benefits, the path to successful integration is not always smooth. Companies often encounter various obstacles that can hinder their progress.
One major challenge is the initial cost of implementing AI technologies. Businesses need to invest in new hardware and software, as well as training for staff. These costs can deter organizations, especially smaller ones, from adopting AI-driven solutions.
Data Quality and Management
Another critical issue is ensuring data quality. AI relies heavily on data to function effectively. Companies must collect, clean, and manage data properly for AI systems to make accurate predictions. Poor data quality can lead to incorrect insights, diminishing the effectiveness of predictive maintenance.
- Inconsistent data formats can create confusion.
- Data silos may prevent comprehensive analysis.
- Outdated information affects decision-making.
- Insufficient data can limit machine learning capabilities.
Additionally, training staff to work with AI technologies presents another hurdle. Employees may need to learn new systems and tools which require time and effort. Organizations that do not invest in employee training risk facing resistance or underutilization of AI technologies.
Cultural Resistance
Resistance to change is also a common barrier. Employees may be hesitant to adopt AI solutions due to fears of job displacement or a lack of understanding about the technology. Those in leadership positions must foster a culture of trust and education to support the integration of AI.
Effective communication about the benefits of AI can help alleviate concerns and encourage acceptance. Companies that focus on education and transparency build a workforce that is more open to change and eager to engage with new technologies.
Finally, organizations must navigate the complexity of AI algorithms. Understanding how these systems make decisions is essential for troubleshooting. If an AI model fails to produce the expected results, teams need to analyze the algorithms, which can be a complicated process.
Future trends in AI and maintenance
Future trends in AI and maintenance are poised to revolutionize the manufacturing landscape. As technology advances, the integration of AI into maintenance practices will become even more pronounced, leading to smarter, more efficient operations.
One significant trend is the increasing use of predictive analytics. Companies will rely more heavily on data-driven insights to anticipate equipment failures before they occur. This shift will optimize maintenance schedules and reduce downtime, making operations smoother.
Integration of IoT and AI
The Internet of Things (IoT) will play a crucial role in the future of AI-driven maintenance. Devices equipped with sensors will continuously collect data on equipment performance. This real-time data will feed into AI systems, allowing for immediate analysis and decision-making. Over time, the synergy of IoT and AI will provide unprecedented visibility into the state of machinery.
- Enhanced monitoring for proactive maintenance
- Connection between different devices and systems
- Improved data collection for better insights
- Real-time alerts for equipment issues
Moreover, advancements in machine learning algorithms will enable AI to learn from past maintenance activities and outcomes. As these algorithms evolve, they will provide even more accurate predictions, allowing organizations to refine their predictive maintenance strategies further.
Automation and Robotics
Another trend is the increased use of automation and robotics in maintenance tasks. AI-driven robots could perform routine maintenance checks and minor repairs autonomously. This not only reduces the burden on human workers but also enhances safety in potentially hazardous environments.
As automation becomes more capable, organizations can expect significant reductions in labor costs and improved efficiency. Employees will be freed up to focus on more complex tasks, leading to heightened productivity overall.
Furthermore, with the rise of AI and machine learning, maintenance training will become more advanced. Workers will utilize virtual reality (VR) and augmented reality (AR) to practice and perfect their skills in a simulated environment. This hands-on training approach can enhance understanding and proficiency, better preparing workers for real-world scenarios.
In summary, the future of AI and maintenance will be marked by continuous innovation. As companies keep embracing these trends, they will likely become more competitive and responsive to market demands and technological changes.
FAQ – Frequently Asked Questions about AI in Predictive Maintenance
What is predictive maintenance?
Predictive maintenance is a strategy that uses data analysis to predict when equipment failures might occur, allowing for timely maintenance.
How does AI improve predictive maintenance?
AI enhances predictive maintenance by analyzing large data sets to identify patterns, leading to more accurate predictions of equipment issues.
What are the main benefits of AI-driven predictive maintenance?
The main benefits include reduced downtime, lower maintenance costs, improved safety, and increased operational efficiency.
What challenges can arise when implementing AI solutions?
Challenges include high initial costs, data quality management, staff training, and resistance to change within the organization.