AI-powered sentiment analysis for brand reputation
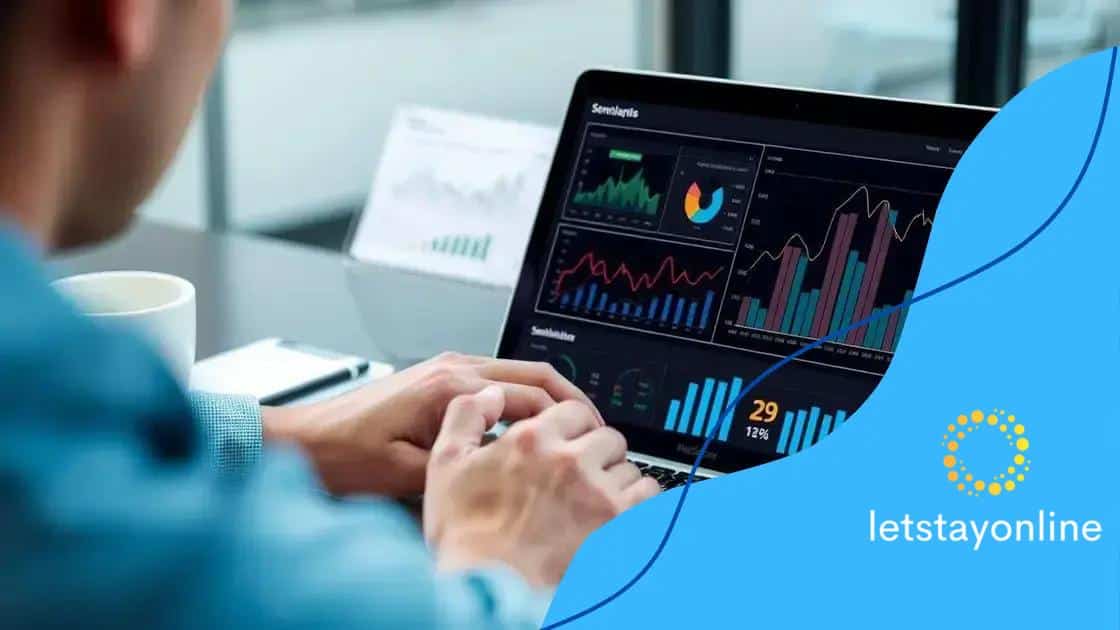
AI-powered sentiment analysis enables brands to understand consumer emotions, enhance customer engagement, and effectively manage their reputation through predictive analytics and tailored strategies.
AI-powered sentiment analysis for brand reputation has become crucial for businesses looking to understand customer opinions. Have you ever wondered how brands gauge public sentiment? This article dives into that intriguing world.
Understanding sentiment analysis and its relevance
Understanding sentiment analysis is key to grasping how brands can interpret public opinion. It’s a process that uses AI to evaluate emotions expressed in text, helping businesses respond appropriately. Not only does it reveal how customers feel about a brand, but it also uncovers trends that might influence future strategies.
The importance of sentiment analysis
Sentiment analysis plays a vital role in today’s digital landscape. With social media and online reviews shaping brand reputation, companies must understand customer feedback to stay competitive. They can capture both positive and negative sentiments, giving valuable insights into customer perceptions.
Key components of sentiment analysis
- Natural Language Processing (NLP) – helps in understanding human language.
- Machine Learning – trains models to classify sentiments accurately.
- Real-time analytics – enables quick responses to customer feedback.
- Data visualization – presents insights in an easily digestible format.
Moreover, businesses can leverage sentiment analysis to enhance customer service. By identifying common complaints or areas of praise, they can adjust their offerings accordingly. This proactive approach not only improves customer satisfaction but also fosters loyalty. Brands that respond to sentiment analysis insights often find themselves at a competitive advantage.
AI-powered sentiment analysis goes beyond simple positive or negative categorizations. It can also assess the intensity of emotions. For instance, a mildly positive review might differ from an ecstatic one. Such nuances help companies fine-tune their messaging and engagement strategies.
Incorporating sentiment analysis into marketing strategies allows brands to tailor their advertising. By understanding how audience sentiment shifts, companies can make informed decisions about campaigns. Employing these insights can lead to more effective communication strategies that resonate with consumers.
How AI enhances sentiment analysis accuracy
Understanding how AI enhances sentiment analysis accuracy showcases the power of technology in improving business insights. By leveraging vast amounts of data, AI can identify patterns that human analysts might miss. This ability to process and analyze text data leads to more precise interpretations of consumer emotions.
Key advancements in AI for sentiment analysis
AI has revolutionized sentiment analysis by employing advanced algorithms. These algorithms are designed to recognize not just the obvious sentiments but also subtle nuances in language. This includes understanding slang, idioms, and contextual meanings that vary in different situations.
Benefits of improved accuracy
- Enhanced customer insights – Brands gain a deeper understanding of public opinion.
- Better decision-making – Accurate sentiment data leads to informed strategic choices.
- Improved customer engagement – Brands can tailor their responses effectively.
- Increased competitive advantage – Companies using AI can outperform competitors who rely on traditional methods.
The incorporation of machine learning is also a game changer. As the system processes more data, it learns to improve its predictions over time. This continuous learning aspect means that the accuracy of sentiment analysis becomes better and more reliable with each interaction.
Real-time processing capabilities allow brands to react promptly to shifts in sentiment. For example, an unexpected negative trend can be addressed immediately, turning potential public relations crises into opportunities for improved customer relations.
Furthermore, the use of natural language processing (NLP) engines enables AI to analyze sentiments in various languages and dialects. This feature is particularly beneficial for global brands that need to understand diverse customer bases.
Use cases of AI-powered sentiment analysis in brands
Use cases of AI-powered sentiment analysis illustrate how brands successfully navigate customer feedback. Many companies leverage this technology to understand their audience better and make informed decisions. For example, brands can monitor social media platforms to gauge public sentiment about launches or marketing campaigns.
Enhancing customer service
AI-powered sentiment analysis enables brands to enhance customer service in meaningful ways. By analyzing feedback from various channels, companies can spot recurring issues swiftly. Knowing what customers feel allows brands to address concerns proactively, leading to increased satisfaction.
Brand monitoring and reputation management
Companies use sentiment analysis for effective brand monitoring. This technology provides insights that help businesses understand how people perceive their brand. By identifying negative sentiments early, they can respond to criticism and mitigate potential damage to their reputation.
- Identifying influencer impact – Brands can see how endorsements affect public opinion.
- Tracking sentiment changes over time – Companies monitor shifts in consumer feelings.
- Engaging with customers – Personalized responses are crafted based on the sentiment analysis outcomes.
In the realm of marketing, AI-powered sentiment analysis offers deep insights into campaign effectiveness. Brands can evaluate reactions to ads, promotions, and events. Understanding whether reactions are positive or negative helps refine future marketing strategies.
Moreover, sentiment analysis helps in product development. When brands analyze customer feedback post-launch, they gain insights into what features work and what needs improvement. This data can inform roadmaps for future product iterations, aligning offerings closely with consumer expectations.
Additionally, sentiment analysis is an effective tool for competitive analysis. By assessing competitors’ public reception, companies can find opportunities to capitalize on or identify gaps they can fill in the market. This strategic advantage is beneficial in shaping product offerings.
Challenges in implementing sentiment analysis effectively
Implementing sentiment analysis effectively presents several challenges that brands must navigate. Despite its benefits, many organizations find it difficult to harness the full potential of this technology. Understanding these challenges is crucial for overcoming them and achieving better results.
Data quality and volume
One major challenge is ensuring high-quality data. Sentiment analysis relies on large datasets to function correctly, and the data must be accurate and relevant. Incomplete or biased data can lead to misleading results. Additionally, the rapidly changing nature of online language adds complexity, as slang and new terms emerge frequently.
Contextual understanding
Another hurdle is the context in which sentiments are expressed. Words can have different meanings based on their usage. For instance, sarcasm can entirely change the sentiment of a statement. Therefore, having an AI system that accurately interprets context is essential for providing reliable insights. This requires continuous training and improvement of algorithms.
- Sentiment varies by audience – Different demographics may perceive the same message differently.
- Customizing sentiment analysis tools can be resource-intensive.
- Monitoring real-time data requires adequate infrastructure.
- Lag in response to emerging trends can lead to missed opportunities.
Moreover, integrating sentiment analysis with existing systems can be complicated. It often requires collaboration between different departments, such as IT and marketing, to ensure that the tools work well together. This integration is vital for making data actionable across the organization.
Furthermore, there is a need for skilled personnel who understand both the technology and the market context. Many companies might face a skills gap when trying to implement these advanced tools effectively. Without the right expertise, harnessing the full potential of sentiment analysis becomes difficult.
Finally, privacy concerns and data regulations must be considered. Companies must ensure that they comply with laws when collecting and analyzing customer data. Failing to address these legal aspects can lead to significant consequences and undermine customer trust.
The future of AI in brand reputation management
The future of AI in brand reputation management looks promising as technology continues to evolve. With companies increasingly relying on data to shape their strategies, AI will play a central role in understanding and managing brand perceptions. As consumer expectations change, brands must adapt quickly to maintain their reputation.
Predictive analytics
One exciting development is the use of predictive analytics. AI systems can analyze past data to forecast future trends and potential reputational risks. By identifying patterns in consumer behavior, brands can take proactive steps to mitigate negative sentiments before they escalate.
Personalized consumer engagement
AI is set to transform how brands engage with consumers. Through sentiment analysis, companies can better understand their target audience’s feelings and preferences. This knowledge allows for highly personalized marketing campaigns that resonate with consumers, improving brand loyalty.
- Dynamic content adjustments based on real-time feedback.
- Tailored responses that reflect individual consumer sentiments.
- Enhanced communication channels that meet customer expectations.
- Strengthened relationships through empathetic customer support.
Furthermore, AI will enhance crisis management strategies. Automated monitoring tools can scan social media and online platforms for mentions of a brand. Quickly identifying negative feedback allows brands to respond promptly, thus preserving their reputation. In an era where information spreads rapidly, timely responses are more crucial than ever.
Another important aspect is ethical AI usage. As brands embrace AI for reputation management, they must also consider ethical implications. Transparency in data use can help maintain consumer trust. Brands need to ensure that their AI systems work fairly and respectfully towards all consumer voices.
Looking ahead, companies that leverage AI-driven insights will likely outperform competitors. The integration of AI in brand reputation management will pave the way for more effective strategies. As technology continues to advance, it is essential for brands to stay updated on these developments to secure their reputation in an ever-changing marketplace.
FAQ – Questions about AI and Brand Reputation Management
How does AI help in brand reputation management?
AI analyzes customer sentiments, helping brands understand how they are perceived and adjust strategies accordingly.
What are the key benefits of using sentiment analysis?
The key benefits include enhanced customer insights, personalized engagement, and improved crisis management strategies.
What challenges do brands face when implementing sentiment analysis?
Brands often struggle with data quality, contextual understanding, and ethical considerations in AI use.
What does the future hold for AI in managing brand reputation?
The future includes advancements in predictive analytics, personalized consumer interactions, and dynamic response strategies to manage reputation effectively.