Explainable AI: Building trust in automated decisions
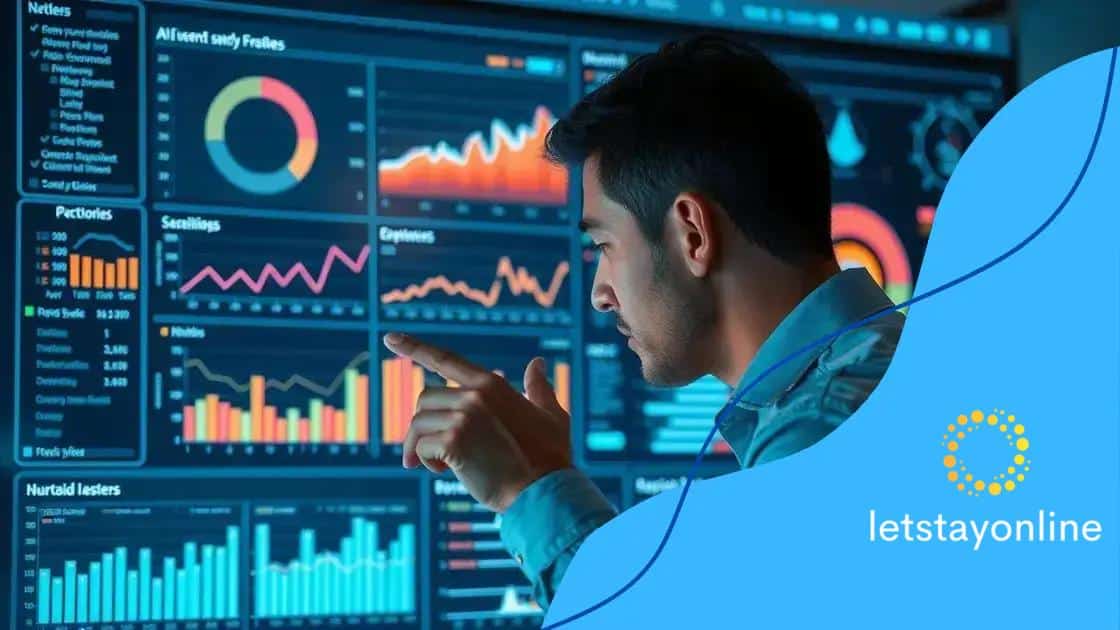
Explainable AI enhances trust in automated decisions by providing clear insights into how AI systems operate, making technology more transparent and user-friendly.
Explainable AI is essential for fostering trust in automated decision-making. As technology advances, understanding how these systems work becomes vital. Ever wondered how your data influences results? Let’s uncover the key elements of this fascinating field.
Understanding explainable AI
Understanding explainable AI is vital for anyone involved in technology today. It’s not just about how algorithms work but also about how their decisions can be understood by users. As automated systems become more integrated into our daily lives, the demand for transparency grows.
What is explainable AI?
Explainable AI refers to methods and techniques in artificial intelligence that make the results of the solution understandable by humans. It contrasts with “black box” models that lack transparency.
Benefits of explainable AI
- Improves trust in automated decisions.
- Enhances user understanding of AI processes.
- Facilitates better troubleshooting and system performance.
When users can see how decisions are made, they are more likely to trust the outcomes. This can lead to better acceptance of AI tools, especially in sensitive areas like finance and healthcare.
Why do we need explainable AI?
As reliance on AI grows, businesses must ensure that their AI systems are transparent. Users often feel uneasy about decisions made by machines, particularly if they don’t understand the reasoning behind them. Explainable AI aims to bridge this gap. Providing insights into algorithms helps mitigate fears and promotes ethical usage of technology.
Moreover, regulatory bodies are beginning to require explanations for automated decisions. Companies that adopt explainable AI will be ahead of the curve in complying with these evolving standards.
Challenges in achieving explainability
Despite its importance, achieving true explainability can be difficult. Some challenges include:
- Complexity of algorithms.
- Trade-offs between accuracy and interpretability.
- The need for ongoing updates and explanations as systems learn.
As researchers continue to tackle these challenges, the landscape of explainable AI will evolve. By emphasizing transparency, we can ensure that automated decisions are not just accurate but also trusted and understood.
Importance of transparency in AI
The importance of transparency in AI cannot be overstated. As artificial intelligence systems make decisions affecting our lives, understanding how these decisions are made becomes crucial. Transparency allows users to see the rationale behind automated decisions, which fosters trust and accountability.
Building trust through transparency
When AI systems are transparent, users feel more comfortable using them. Knowing the logic behind a decision helps people understand outcomes better. This is especially important in fields like healthcare and finance, where decisions can have significant impacts on lives and well-being.
Benefits of transparency in AI
- Enhances user confidence in AI systems.
- Encourages ethical AI development.
- Facilitates compliance with regulations.
Transparency also encourages ethical practices in AI development. When companies are open about their algorithms and decision-making processes, they are more likely to be held accountable for their actions. This openness can also lead to better collaboration between stakeholders, resulting in improved system design and performance.
Challenges to achieving transparency
Despite its benefits, achieving transparency in AI is challenging. Many AI models involve complex algorithms that are hard to interpret. There is often a tension between the accuracy of these models and their interpretability. Users may need clearer explanations of how decisions are reached.
Moreover, as AI systems evolve and are updated, maintaining transparency can become even more difficult. It is essential for developers to prioritize clarity and accessibility in their explanations. This will help bridge the gap between advanced algorithms and user understanding, ensuring responsible AI usage.
Building trust through explainability
Building trust through explainability is critical in today’s AI-driven world. Users need to understand how decisions are made by these systems to feel secure. When decisions come from a “black box,” it can lead to skepticism and hesitance in adopting new technologies.
How explainability fosters trust
Explainability helps users see the logic behind AI decisions. When people can understand the factors and data that lead to a conclusion, they are more likely to trust the process. For instance, if an AI system in healthcare suggests a treatment plan, knowing the basis for that recommendation helps patients feel comfortable with their options.
Elements that enhance trust
- Clear communication of decision-making processes.
- Accessible explanations tailored to users’ needs.
- Real-time feedback on AI workings.
Accessible explanations can vary from simple summaries to complex data breakdowns. This adaptability allows users from different backgrounds to engage with the information effectively. The more transparent the process, the stronger the trust built between users and the AI.
Real-world applications
In industries such as finance and healthcare, establishing trust is vital. For example, lending decisions made by AI should provide insights into credit scoring. Clients who can see how their scores are calculated can better understand and accept the outcomes. This transparency can also lead to improved decision-making and accountability.
Furthermore, organizations that implement explainability strategies can enhance user satisfaction and loyalty. By prioritizing clear communication and understanding, companies can cultivate a strong relationship with their audience. Ultimately, this leads to a more positive perception of AI tools.
Challenges in implementing explainable AI
Challenges in implementing explainable AI are significant but essential to address. As organizations strive to integrate AI systems, understanding and overcoming these hurdles is crucial for effective deployment. Many developers face obstacles related to complexity and transparency.
Complexity of AI models
One major challenge is the inherent complexity of AI algorithms. Many models operate as “black boxes,” making it hard to extract understandable reasoning behind their decisions. For example, deep learning networks, while powerful, often lack clear explanations of how they process data.
Balancing accuracy and interpretability
Another issue is the trade-off between accuracy and interpretability. More accurate models may use complex structures that provide little insight into their workings. This dilemma poses a significant challenge for developers who want both reliable performance and clear explanations.
- Complexity can obscure decision-making paths.
- Accurate models often require intricate algorithms.
- Efforts to simplify may reduce effectiveness.
Moreover, as AI systems evolve and learn, maintaining transparency can become even more challenging. Organizations must invest in tools and strategies that allow ongoing evaluation of their models to ensure clarity remains a priority throughout the system’s lifecycle.
Cost and resource considerations
Implementing explainability features can also be resource-intensive. Organizations might face financial constraints that limit the ability to develop and integrate required tools. This might slow down the deployment of more ethical and trustworthy AI technologies.
Furthermore, training personnel to understand and manage these explainability tools can require additional time and resources. Staff need ongoing education and support to navigate the complexities of AI models effectively. This learning curve can further complicate the implementation process of explainable AI.
Future trends in explainable AI
Future trends in explainable AI promise exciting advancements that can enhance the way we understand automated systems. As organizations recognize the importance of trust and transparency, several key trends are emerging in this field.
Enhanced interpretability methods
One major trend involves the development of more sophisticated interpretability methods. Researchers are working on creating models that not only achieve high accuracy but also provide clear explanations for their outputs. This dual focus can lead to broader adoption of AI technologies across various industries.
Integration of user feedback
Another significant development is the integration of user feedback into AI systems. By incorporating insights from users, developers can create models that are more attuned to the needs and expectations of their audiences. This not only improves user satisfaction but also makes AI systems more relevant.
- User-centric design will shape explainability tools.
- Feedback loops will enhance learning processes.
- Greater customization will meet specific user needs.
These feedback-driven approaches help refine the models over time, ensuring they maintain relevance and effectiveness as user needs evolve. This dynamic relationship between AI systems and users can promote ongoing trust and reliability.
Regulatory developments
Additionally, as regulations around AI continue to evolve, there will be a stronger emphasis on explainability. Future policies may mandate clearer standards for how AI systems disclose their decision-making processes. This means that organizations will need to adapt quickly to comply with new laws, which can drive further advancements.
As a result, companies that proactively develop their explainability strategies will not only be better positioned to meet regulatory requirements but will also cultivate a competitive advantage. The trend toward stricter regulations reflects society’s growing expectations for accountability in AI.
In conclusion, the future of explainable AI is bright and full of potential. As technology continues to evolve, organizations must prioritize transparency to build trust with users. By focusing on the challenges and implementing strategies that enhance interpretability, AI systems can meet the growing demand for ethical uses of technology. The integration of user feedback and adherence to emerging regulations will further shape this evolution, making explainable AI not just a goal, but a standard practice across industries. Embracing these trends will ensure AI systems not only serve their purposes effectively but also create a positive relationship with the people who use them.
FAQ – Frequently Asked Questions about Explainable AI
Why is explainability important in AI systems?
Explainability is crucial because it helps build trust with users, allowing them to understand how decisions are made and feel more comfortable using AI technologies.
What are the main challenges in implementing explainable AI?
Key challenges include the complexity of algorithms, balancing accuracy with interpretability, and the costs associated with developing explainability features.
How can user feedback improve explainable AI?
Integrating user feedback helps developers refine models and ensure the AI systems are relevant to users’ needs, enhancing satisfaction and trust.
What future trends can we expect in explainable AI?
Future trends may include improved interpretability methods, stricter regulations requiring clearer explanations, and greater focus on user-centric designs.