Leveraging data lakes for business intelligence success
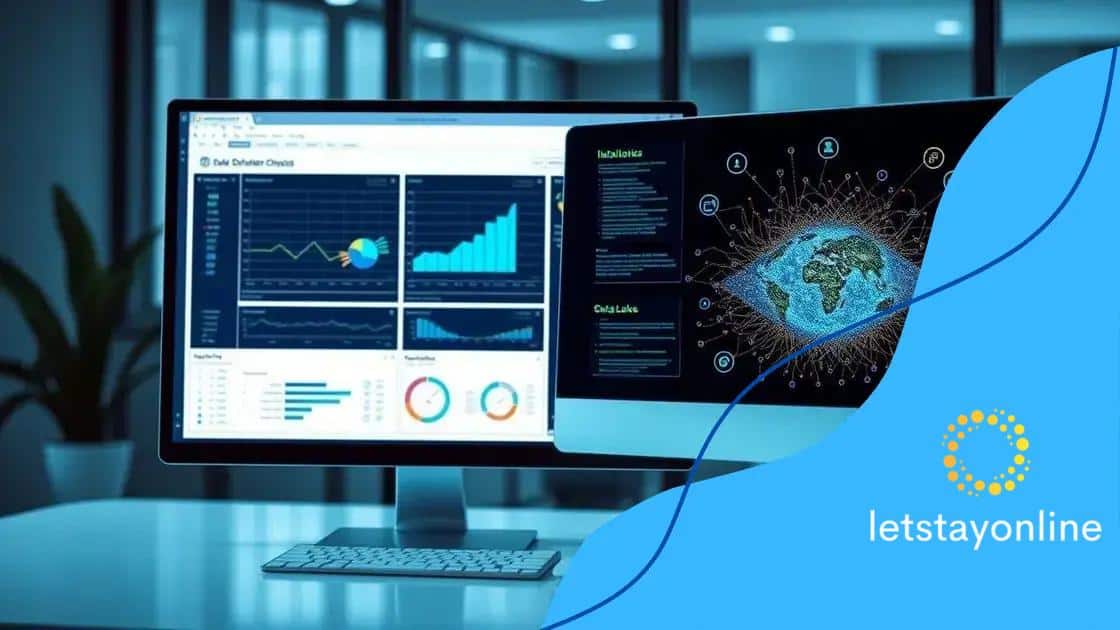
Leveraging data lakes for business intelligence allows organizations to consolidate diverse data sources, enhance analytics, and drive informed decision-making through improved data accessibility and collaboration.
Leveraging data lakes for business intelligence opens up new avenues for informed decision-making. Have you ever wondered how organizations are managing massive datasets effectively? In this article, we’ll dive into the essentials.
What are data lakes and why do they matter?
Data lakes are essential storage repositories that hold vast amounts of raw data in its native format until it’s needed. In today’s digital landscape, understanding what data lakes are and why they matter is crucial for any business aiming to leverage data effectively.
This approach offers flexibility. Companies can store structured, semi-structured, and unstructured data all in one place. This versatility enables businesses to analyze data without having to first convert it into a specific model. Think of a data lake as a large reservoir where all types of data flow in, ready for insights whenever needed.
Key Characteristics of Data Lakes
Understanding the key characteristics of data lakes is vital for leveraging their capabilities. Here are some noteworthy aspects:
- Scalability: Data lakes can grow easily, accommodating increasing volumes of data.
- Cost-Effectiveness: Storing data in its raw form can be cheaper than traditional database solutions.
- Flexibility: Businesses can quickly adapt to changing data needs without significant upfront investments in data infrastructure.
The importance of data lakes cannot be overstated. They provide a powerful foundation for advanced analytics, machine learning, and business intelligence initiatives. Companies can derive meaningful insights from massive datasets that were previously hard to analyze.
Moreover, data lakes support a wide range of analytical tools and processing frameworks. This adaptability allows organizations to tailor their analyses based on their specific needs, whether it’s for real-time processing or historical data analysis.
In conclusion, recognizing what data lakes are and their significance in today’s data-driven environment can provide companies with a competitive edge. They enable better decision-making, foster innovation, and streamline processes, making them a critical component of modern data strategies.
Benefits of leveraging data lakes for analytics
Leveraging data lakes for analytics provides numerous advantages for businesses today. Understanding these benefits is essential for any organization aiming to enhance its decision-making processes. Data lakes enable seamless access to a vast array of data, allowing companies to harness insights effectively.
This approach enhances collaboration across various teams. Data scientists, analysts, and business users can access the same datasets, facilitating teamwork and improving communication. As they dive into data together, the potential for innovation increases significantly. The ability to analyze data in real-time further amplifies this effect, allowing teams to adapt quickly to changing conditions.
Key Benefits of Data Lakes for Analytics
Here are some key benefits of leveraging data lakes for analytics:
- Cost Efficiency: Storing large volumes of data in data lakes is often less expensive than traditional data warehouses.
- High Scalability: Data lakes can easily grow with your organization’s needs, accommodating any data influx.
- Enhanced Flexibility: Companies can store and analyze different types of data without any need for complex data modeling.
This flexibility allows for various analytic use cases. Organizations can perform complex queries, run machine learning models, or even conduct exploratory data analysis. As businesses become more data-driven, the need for such capabilities continues to rise.
Data lakes also support unstructured data types, such as videos, images, and social media posts. By leveraging these diverse data points, companies can gain a more comprehensive understanding of their operations and customer behaviors.
As businesses focus on leveraging data lakes for analytics, they can uncover trends and insights that drive better business strategies. This proactive approach sets businesses up for long-term success in their respective markets.
Steps to integrate data lakes in your business
Integrating data lakes into your business can transform how you manage and analyze data. Following structured steps makes this process efficient and effective. The journey starts with understanding your organization’s data needs and how a data lake can meet those needs.
The first step involves assessing your current data architecture. Identify where your data is stored, how it flows, and what formats it is in. This assessment will help you pinpoint gaps and opportunities to leverage a data lake. It’s essential to engage stakeholders from different departments to gather comprehensive insights.
Defining Your Data Strategy
Creating a clear data strategy is vital. This strategy should address how your organization plans to collect, store, and utilize data. Here are some important components:
- Data Sources: Identify all the sources of data you want to integrate into the data lake.
- Data Types: Determine the types of data (structured, unstructured) you will be working with.
- Compliance Needs: Consider any regulatory or compliance requirements your data must adhere to.
Once your data strategy is in place, the next step is selecting the right technology. Numerous platforms in the market support data lake operations. It’s crucial to choose one that aligns with your business goals and technical requirements. Look for features like scalability, security, and ease of use.
After selecting a platform, focus on data ingestion. Decide how you will move data from various sources into your data lake. This process may involve designing ETL (Extract, Transform, Load) pipelines that streamline data flow. Automation can also be beneficial to ensure timely updates to your data lake.
Finally, set up governance and management frameworks. Establish clear processes for managing and accessing data within the lake. This includes defining roles, permissions, and data quality standards. Ensuring data security is paramount, so incorporating robust security measures will protect sensitive information.
Best practices for data governance in data lakes
Implementing strong data governance in data lakes is crucial for ensuring data integrity and security. Businesses must establish clear policies and procedures to manage data effectively. Without proper governance, organizations may face challenges such as data breaches or compliance issues.
One of the first steps in data governance is defining roles and responsibilities. Ensure everyone involved understands their tasks and the importance of data management. This clarity helps create a culture of accountability across the organization.
Key Components of Data Governance
Here are some key components to focus on:
- Data Quality: Regularly monitor and assess data quality to ensure accuracy and reliability of information.
- Access Control: Implement strict access controls to protect sensitive data. Only authorized personnel should access certain datasets.
- Compliance: Stay updated on relevant regulations and ensure that data practices align with these requirements.
Establishing data stewardship is also vital. Appoint data stewards to oversee critical data assets. These individuals are responsible for maintaining data quality, resolving data issues, and ensuring that data policies are followed. They act as a bridge between technical teams and business users, enhancing collaboration.
Regularly reviewing and updating governance policies is necessary as data practices and technology evolve. Create a schedule for frequent assessments to adapt to new challenges or regulatory changes. Document any changes made to maintain transparency and track decisions over time.
Finally, invest in training and education for your team. Enhancing their understanding of data governance fosters a culture of responsibility and informed data use. By equipping employees with the tools and knowledge they need, organizations can improve data handling and ensure compliance effectively.
Real-world examples of successful data lake implementations
Real-world examples of successful data lake implementations showcase how businesses transform their operations using data strategically. Companies across various industries have harnessed the power of data lakes to drive insights and innovation.
One impressive example comes from a leading retail company. They integrated a data lake to consolidate data from various sources, including point-of-sale systems and online platforms. By doing so, they streamlined their data analysis processes, improved inventory management, and personalized customer experiences. The results were significant, with a reported increase in sales through targeted marketing strategies.
Healthcare Industry
In the healthcare sector, a prominent hospital network implemented a data lake to collect patient data from electronic health records, wearable devices, and lab results. This integration allowed doctors to access comprehensive patient histories quickly. Improved data accessibility helped in making faster diagnosis and enhanced patient care, ultimately leading to better health outcomes.
- Data-Driven Decisions: The hospital could analyze trends in patient data, leading to proactive treatment plans.
- Cost Efficiency: By reducing unnecessary tests and procedures, the hospital saved on operational costs.
- Enhanced Collaboration: Different departments accessed the same data, improving communication among healthcare professionals.
Another notable case is an energy company that adopted a data lake to monitor equipment performance in real time. By analyzing data from sensors and maintenance records, they proactively identified potential equipment failures. This predictive maintenance strategy reduced downtime and ensured operational efficiency.
The manufacturing sector has also seen benefits from data lakes. A manufacturing firm collected data from its machines, production lines, and supply chains. With this data, they optimized production schedules and reduced waste. The ability to visualize data trends made it easier to streamline operations, which boosted overall productivity.
These examples illustrate how organizations are successfully leveraging data lakes to advance their business goals. By utilizing large volumes of data effectively, companies can not only improve efficiencies but also create innovative solutions tailored to their specific challenges.
FAQ – Frequently Asked Questions about Leveraging Data Lakes for Business Intelligence
What is a data lake and how does it differ from a data warehouse?
A data lake is a storage repository that holds vast amounts of raw data in its native format, while a data warehouse stores processed and structured data specifically for analysis.
What are the key benefits of using data lakes for analytics?
Data lakes provide cost efficiency, high scalability, and enhanced flexibility, allowing organizations to analyze various types of data without upfront modeling requirements.
How can data governance be implemented effectively in data lakes?
Data governance can be established by defining roles, ensuring data quality, implementing access controls, and regularly reviewing policies to meet evolving compliance needs.
Can you provide an example of a successful data lake implementation?
A leading retailer used a data lake to consolidate data from various sources, which improved inventory management and personalized customer experiences, leading to increased sales.