Predictive analytics for optimizing ad spend effectively
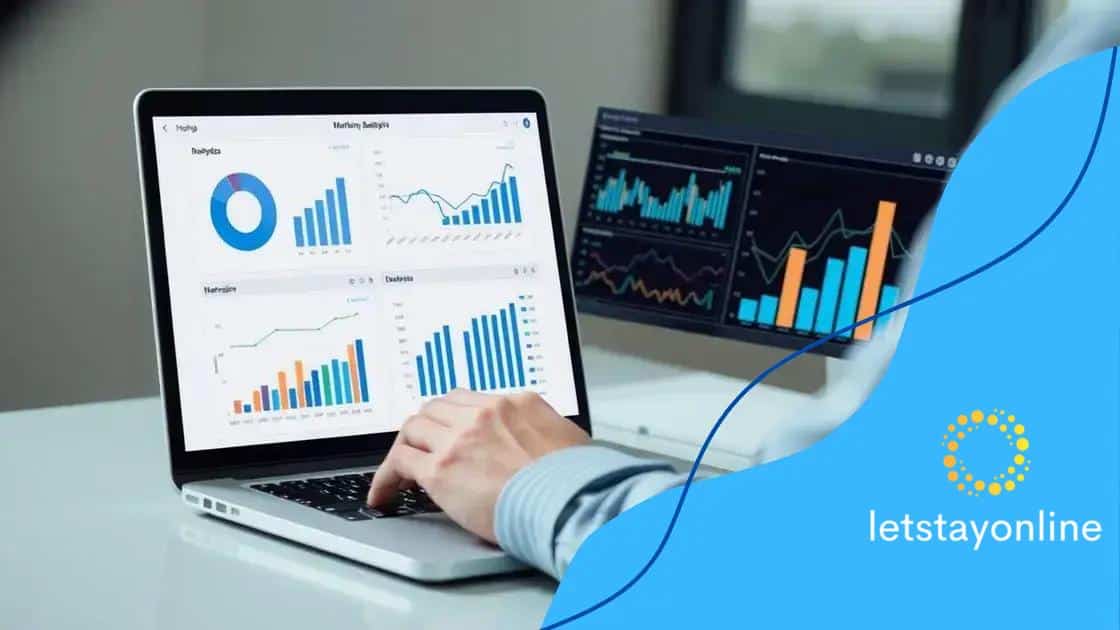
Predictive analytics for optimizing ad spend enables businesses to make data-driven decisions, improving targeting, reducing costs, and enhancing overall advertising effectiveness through insights derived from past performance and consumer behavior.
Predictive analytics for optimizing ad spend is becoming a game changer for marketers aiming to enhance their advertising efforts. Have you ever wondered how data can inform your spending decisions? This article dives into the essentials of predictive analytics and its growing importance.
Understanding predictive analytics in advertising
Understanding predictive analytics in advertising is crucial for advertisers who want to make informed decisions. This approach utilizes data to forecast trends and outcomes, helping businesses optimize their ad spend.
By analyzing past performance, advertisers can identify which campaigns were successful and why. This insight allows them to allocate resources more effectively in future campaigns.
Key Concepts of Predictive Analytics
Predictive analytics relies on various methodologies, including statistical modeling and machine learning. These techniques provide insights that are not easily visible through traditional analysis.
- Data collection: Gathering data from multiple sources is essential for robust predictive models.
- Data processing: Cleaning and organizing data helps in accurate analysis and predictions.
- Modeling: Building predictive models involves selecting algorithms that best fit your data.
Once a model is developed, it can be used to simulate different scenarios. For instance, businesses can predict how changes in ad spend might impact overall sales. This ability to foresee results before making changes allows for a proactive approach rather than a reactive one.
Benefits of Utilizing Predictive Analytics
Implementing predictive analytics can lead to numerous advantages. Companies can enhance their decision-making process, increase efficiency, and ultimately maximize return on investment (ROI).
- Improved targeting: Ads can be tailored to specific audiences, leading to higher engagement rates.
- Cost reduction: By focusing on the most effective strategies, companies can save money while improving results.
- Data-driven decisions: Businesses rely on concrete data rather than gut feelings, promoting more accurate outcomes.
Moreover, using predictive analytics allows businesses to stay ahead of trends. They can identify emerging patterns in consumer behavior, ensuring their campaigns remain timely and relevant. As the advertising landscape evolves, those who adapt quickly will thrive.
In conclusion, predictive analytics in advertising not only streamlines the ad spend process but also empowers marketers to make smarter, data-informed decisions. Embracing this technology can transform an average advertising strategy into a highly successful one, development that is essential for staying competitive in today’s market.
Key benefits of predictive analytics for ad spend
Key benefits of predictive analytics for ad spend are numerous and impactful. Businesses that embrace this technology can gain a competitive edge in their advertising efforts.
One of the significant advantages is the ability to maximize return on investment (ROI). By leveraging data, advertisers can pinpoint which advertisements perform best, allowing them to allocate budgets more effectively.
Enhanced Decision-Making
Predictive analytics provides insights that help marketers make informed choices. For instance, with data analysis, advertisers can understand audience preferences and behaviors. This understanding aids in crafting more effective campaigns.
- Data-driven insights improve targeting precision.
- Identify trends before they emerge, capitalizing on market movements.
- Resource allocation becomes smarter, reducing waste.
Moreover, utilizing predictive analytics helps in optimizing ad spend by guiding strategic decisions. Instead of guessing which ad will perform better, advertisers can follow evidence from previous campaigns, leading to more successful outcomes.
Cost Efficiency
Implementing predictive analytics also enables cost efficiency. Companies can assess various scenarios before launching a campaign, helping to avoid unnecessary expenditures. By analyzing past data, they can see which strategies yielded the best results and apply those lessons to new projects.
- Invest in high-performing channels, reducing costs on ineffective ones.
- Test campaigns in a virtual environment to simulate outcomes.
- Regularly update models to adapt to changing consumer behavior.
Furthermore, the ability to fine-tune campaigns in real-time is a game changer. If something isn’t working, marketers can pivot quickly based on data insights. This agility ensures that every advertising dollar is spent wisely, making a significant difference in overall campaign performance.
In sum, the benefits of predictive analytics for ad spend not only streamline the advertising process but also empower marketers to be proactive and data-driven in their strategies. Embracing this technology can lead to improved performance, cost savings, and overall success in advertising efforts.
How to implement predictive analytics models
How to implement predictive analytics models is a crucial step for businesses seeking to optimize their advertising strategies. Understanding the process can greatly enhance your decision-making.
To start, gather relevant data from various sources. This data can include past ad performance, audience demographics, and market trends. The quality of data is essential as it directly impacts the accuracy of the models built.
Steps to Implement Predictive Analytics
Once you have collected data, the next steps can be broken down into clear phases:
- Data Cleaning: Remove any inaccuracies and irrelevant information from your dataset.
- Data Analysis: Explore the data using statistical techniques to identify patterns and relationships.
- Model Selection: Choose the appropriate predictive model based on your goals, whether it’s regression, decision trees, or machine learning algorithms.
After choosing the model, it’s time to train it using historical data. This involves feeding the model with data it can learn from, refining its ability to make predictions. The model will generate outputs based on the input data, which can help you forecast future trends.
Testing and Validation
Next, validate the model’s performance by testing it with new data. This step is vital to ensure that it predicts accurately. Use metrics such as Root Mean Squared Error (RMSE) or accuracy percentages to assess how well the model performs.
- Test datasets should be distinct from training datasets to avoid overfitting.
- Adjust parameters and refine the model based on testing results.
- Continuously monitor the model’s performance over time to ensure its effectiveness.
As you implement predictive analytics, remember that this is an ongoing process. Regularly update your models with fresh data, as market conditions and consumer behaviors change. The more you adapt, the more sharp and relevant your predictions will become.
Incorporating these strategies can significantly enhance how you manage and optimize your ad spend through data-driven insights.
Case studies showcasing successful ad spend optimization
Case studies showcasing successful ad spend optimization provide valuable insights into how businesses effectively use predictive analytics. These examples illustrate the impact of data-driven strategies on advertising effectiveness.
One compelling case study comes from a major retail brand that implemented predictive analytics to refine their targeting. By analyzing customer data, they found that certain demographics responded better to specific promotions. As a result, they adjusted their ad spend to focus more on these audience segments, leading to a significant increase in conversion rates.
Real-World Examples
In another instance, a technology company utilized predictive models to assess the effectiveness of their digital marketing campaigns. They discovered that ads running during peak hours yielded better engagement. By reallocating their budget to prioritize these times, they improved overall ad performance and reduced unnecessary spending.
- Retail Brand: Enhanced targeting increased conversion rates by 25%.
- Technology Company: Optimized budget allocation led to a 30% rise in engagement.
Additionally, a travel agency leveraged predictive analytics to identify trends in seasonal travel behavior. With this insight, they ran targeted campaigns leading up to peak travel seasons. This strategy not only maximized their ad spend but also resulted in a notable increase in bookings compared to previous years.
Key Takeaways
These case studies emphasize the importance of being data-informed. Businesses can drastically improve their outcomes by employing predictive analytics to guide ad spend decisions. Adopters of this approach report greater efficiency, higher ROI, and more meaningful engagement with their target audiences.
- Using data to drive decisions is crucial for optimizing ad spend.
- Tailoring campaigns to specific demographics yields better results.
- Timing and seasonality are critical factors in campaign effectiveness.
Such examples of successful ad spend optimization highlight the transformative power of predictive analytics and its growing importance across various industries.
Future trends in predictive analytics for advertising
Future trends in predictive analytics for advertising promise to reshape how companies engage with their audiences. As technology evolves, so do the strategies advertisers use to optimize their spending.
One emerging trend is the increased use of artificial intelligence (AI) in predictive analytics. AI can process large volumes of data quickly, enabling advertisers to gain insights at a faster pace. This allows businesses to adapt their strategies in real-time, ensuring they remain competitive.
Enhanced Personalization
Another significant trend is the move towards hyper-personalization. As data collection becomes more sophisticated, companies are expected to create tailored experiences for consumers. By leveraging predictive analytics, businesses can analyze individual customer behaviors and preferences, making their marketing efforts far more relevant.
- Utilizing customer segmentation for targeted campaigns.
- Delivering personalized content based on past interactions.
- Predicting future shopping behavior for better ad placements.
Moreover, predictive analytics is expected to integrate more seamlessly with other marketing technologies. This integration will enhance cross-channel strategies, allowing companies to harmonize their advertising efforts across various platforms.
Data Privacy and Ethics
As data collection and predictive capabilities advance, concerns regarding data privacy will increase. Advertisers will need to balance their strategies with ethical considerations. Following regulations and respecting consumer privacy will be crucial in building trust and maintaining brand integrity.
- Implementing transparent data usage policies.
- Ensuring compliance with data protection laws.
- Encouraging ethical data collection methods.
Furthermore, advancements in machine learning algorithms will continue to enhance accuracy in predictions. These more sophisticated models will refine the way campaigns are analyzed, allowing for better forecasting and insight generation. As businesses adopt these technologies, they will likely achieve higher ROI on their advertising budgets.
Overall, the future of predictive analytics in advertising looks promising. With the right tools and strategies, companies can unlock new opportunities for growth and engagement with their customers.
FAQ – Frequently Asked Questions about Predictive Analytics in Advertising
What is predictive analytics in advertising?
Predictive analytics in advertising uses data and statistical algorithms to predict future outcomes, helping businesses optimize their ad spending.
How does AI enhance predictive analytics?
AI processes large amounts of data quickly, allowing for faster insights and real-time adjustments to advertising strategies.
Why is hyper-personalization important?
Hyper-personalization tailors ads to individual customer preferences, improving engagement and conversion rates by making marketing efforts more relevant.
How can businesses ensure ethical data usage?
Businesses should implement transparent data policies and comply with regulations to respect consumer privacy and build trust.