Reinforcement learning transforming robotics and automation
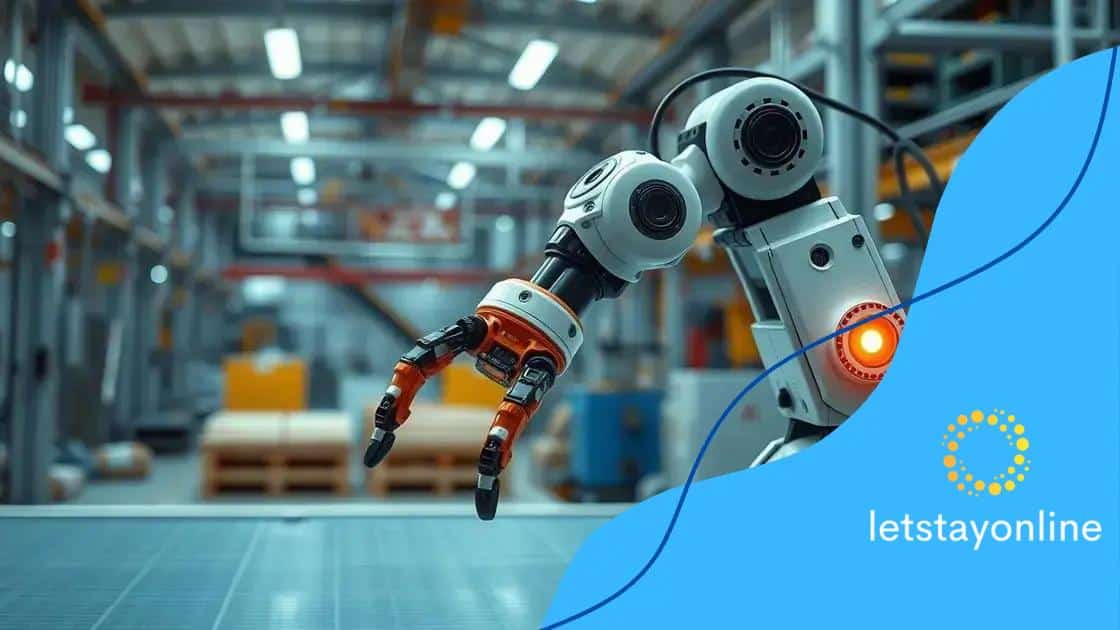
Reinforcement learning is transforming robotics and automation by enabling machines to learn from experiences, optimize their actions through rewards, and adapt to complex tasks in various industries.
Reinforcement learning transforming robotics and automation is changing the game for how machines learn and adapt. Have you ever wondered how these advancements are making robots smarter? Let’s dive in!
Understanding reinforcement learning in robotics
Understanding reinforcement learning in robotics is essential for grasping the revolutionary changes in how machines operate. With this technology, robots can learn from their interactions with the environment, making decisions that improve their tasks over time.
This process is similar to how humans learn—by trial and error. As robots receive feedback on their actions, they adjust their strategies. Let’s explore how this works.
The basics of reinforcement learning
At its core, reinforcement learning involves an agent—like a robot—learning to achieve a goal in an uncertain environment. It uses a system of rewards and penalties to guide learning. Some key components include:
- Agent: The robot or system that is learning.
- Environment: The world in which the agent operates.
- Actions: Choices made by the agent.
- Rewards: Feedback from the environment based on actions taken.
These elements work together to help the agent improve its performance over time.
How reinforcement learning is applied in robotics
In practical applications, reinforcement learning allows robots to adapt to complex tasks, like navigating obstacles or assembling products. For instance, a robot can learn to optimize its path in a warehouse, minimizing time spent moving from point A to point B. This adaptability leads to enhanced efficiency and productivity.
Furthermore, reinforcement learning contributes to developing autonomous systems. These systems can function without constant human input, making decisions based on their learned experiences. Think about self-driving cars—they continuously learn and improve their driving strategies.
Benefits of using reinforcement learning
The advantages of employing reinforcement learning in robotics are significant:
- Increased efficiency: Robots become faster and more effective in their tasks.
- Adaptability: Systems can adjust to new challenges without requiring extensive reprogramming.
- Continuous improvement: As robots learn, their performance continues to enhance.
Understanding reinforcement learning enables us to harness its power fully. This remarkable technology is bridging the gap between human and machine learning, transforming industries and enhancing automation.
Key breakthroughs in automation through RL
Key breakthroughs in automation through RL have reshaped how industries operate. With advancements in reinforcement learning, systems can learn complex processes, leading to smarter automation.
These breakthroughs allow machines to optimize their performance based on feedback. In sectors like manufacturing, robots can now make real-time adjustments, improving production efficiency. Let’s explore some significant advancements that demonstrate this change.
Enhanced decision-making abilities
One major breakthrough is the ability of machines to make better decisions quickly. Through reinforcement learning, robots analyze data and outcomes, allowing them to adjust their actions accordingly. For instance, self-driving cars utilize this technology to determine the best route and avoid obstacles, adapting to real-time traffic conditions.
Applications in various industries
Reinforcement learning has found its way into numerous fields. Here are a few examples:
- Manufacturing: Robots can learn to assemble products more efficiently.
- Healthcare: Automation helps in treatment personalization by learning patient responses.
- Logistics: Systems optimize supply chains by predicting demand patterns.
Such applications have led to significant cost savings and improved quality in service delivery.
Reduction in human intervention
Another significant aspect of breakthroughs through reinforcement learning is the reduction of human involvement in routine tasks. As systems advance, the need for constant supervision diminishes. For instance, automated warehouses use RL algorithms to operate efficiently without needing human operators on-site. This not only saves time but also reduces labor costs.
By continuously learning from their environment, machines evolve, leading to enhanced capabilities that were not possible before. Industries adopting these innovations are witnessing major transformations, paving the way for a future where intelligent automation becomes the norm.
Real-world applications of reinforcement learning
Real-world applications of reinforcement learning showcase how this powerful technology enhances various industries. By enabling machines to learn from experience, reinforcement learning is driving innovation across many fields.
One impactful application is in robotics. Robots utilize reinforcement learning to improve their precision and adaptability when performing tasks. For example, in assembly lines, robots learn to adapt their movements for efficiency, which helps save time and reduce errors.
Finance sector advancements
In finance, reinforcement learning algorithms help predict market trends and optimize trading strategies. This technology analyzes vast amounts of historical data to make informed decisions. Traders use RL models to develop systems that adapt to market changes, maximizing profits while minimizing risks.
Healthcare improvements
Another impressive application is in healthcare. Reinforcement learning aids in treatment personalization by analyzing patient responses. Automated systems can suggest treatments based on individual patient data, improving outcomes and enhancing care.
Additionally, robotic surgeries have seen improvements due to RL, allowing surgeons to perform precise actions based on real-time feedback.
Gaming and entertainment
Reinforcement learning has also made a huge impact in gaming. Machine learning agents learn to play video games by experimenting and adjusting strategies based on success rates. Games like AlphaGo illustrate how RL has advanced to a level where machines can outperform humans in complex games.
This capability not only entertains but also provides insights into strategy development that can be applied in other fields.
By implementing reinforcement learning in these real-world scenarios, industries are transforming, showcasing the significant benefits of this innovative technology.
Challenges and limitations of reinforcement learning
Challenges and limitations of reinforcement learning are important to consider as we explore this advanced technology. While reinforcement learning has incredible potential, it also faces significant hurdles that can limit its effectiveness.
One major challenge is the amount of data required for training. Reinforcement learning algorithms need extensive datasets to learn effectively. This can be a barrier, especially in environments where data is scarce or difficult to obtain. Additionally, training can take a long time, requiring significant computational resources.
Exploration vs. exploitation
Another key challenge is the balance between exploration and exploitation. In reinforcement learning, an agent must decide whether to explore new actions or exploit known ones for rewards. This trade-off is critical because excessive exploration can lead to inefficient learning, while too much exploitation can prevent discovering better strategies.
Overfitting and generalization
Overfitting is another issue that arises during the training process. When reinforcement learning models focus too much on the training environment, they may fail to perform well in new situations. This lack of generalization can hinder the model’s ability to adapt to real-world challenges.
Moreover, the specific design of the reward structure significantly impacts performance. If the rewards are not structured properly, it can lead to unintended behaviors in the agent. Aligning rewards with desired outcomes is essential for successful reinforcement learning applications.
Ethical considerations
Ethical concerns also play a role, particularly when deploying reinforcement learning in high-stakes environments. Decisions made by RL algorithms can have significant consequences, and there is a need for transparency in how these systems operate. Ensuring that RL agents behave in a safe and responsible manner is crucial.
Overall, despite its challenges, understanding the limitations of reinforcement learning is key to developing more effective applications. By addressing these issues, we can harness the full potential of this powerful technology.
Future trends in robotics and automation
Future trends in robotics and automation promise exciting developments that will reshape industries. As technology advances, the integration of artificial intelligence (AI) and machine learning into robotics is becoming more sophisticated.
One strong trend is the increasing collaboration between humans and robots. Collaborative robots, or cobots, are designed to work alongside humans, enhancing productivity without replacing human jobs. These machines can learn from human actions, making them more adaptable and efficient in diverse environments.
Autonomous systems
Autonomous systems are another key area of growth. Future robots will operate with minimal human intervention, making decisions based on real-time data. This is particularly important in sectors like agriculture, where autonomous drones can monitor crops and optimize yields.
Enhanced AI integration
The integration of advanced AI will lead to smarter robots that can learn from their mistakes. Using techniques like reinforcement learning, robots can improve their performance over time. This capability allows them to handle complex tasks that were once too challenging for machines.
Moreover, the improvements in AI will enhance robots’ interaction with their environments. They will be able to recognize objects and respond to human emotions, creating more intuitive and user-friendly systems.
Focus on sustainability
Another emerging trend is the focus on sustainability. Future robotics will emphasize energy efficiency and eco-friendly practices. Innovations in battery technology and energy harvesting will allow robots to operate longer and with less environmental impact. For example, solar-powered robots could help reduce reliance on traditional energy sources.
As these trends develop, we will also see increased investment in research and development. Governments and private companies are eager to explore how robotics can solve real-world problems, from healthcare to environmental conservation.
The future of robotics and automation is bright, filled with potential that can transform our world for the better.
In conclusion, the future of robotics and automation is exciting and full of possibilities. As technology continues to advance, we can expect to see smarter machines that work alongside humans. With better AI integration, autonomous systems, and a focus on sustainability, these trends will transform industries and improve lives. While there are challenges to overcome, the potential benefits of leveraging reinforcement learning in robotics are immense. Together, we can look forward to a brighter, more efficient future.
FAQ – Frequently Asked Questions about Reinforcement Learning in Robotics and Automation
What is reinforcement learning?
Reinforcement learning is a type of machine learning where an agent learns to make decisions by receiving feedback from its environment in the form of rewards or penalties.
How do robots use reinforcement learning?
Robots use reinforcement learning to improve their performance over time by learning from their experiences, allowing them to adapt to new tasks and environments.
What are some real-world applications of reinforcement learning?
Applications include autonomous vehicles, robotics in manufacturing, personalized healthcare, and even gaming strategies, where machines learn and adapt through trial and error.
What are the main challenges in applying reinforcement learning?
Challenges include the need for large amounts of training data, balancing exploration and exploitation, and ensuring that models generalize well to new situations.